Viewpoints
Powering the next phase of life sciences innovation
Q&A with Dr.Evidence’s Chief Innovation Officer, Umut Eser, on reversing the challenges of drug discovery and powering the next leap forward in innovation
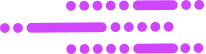
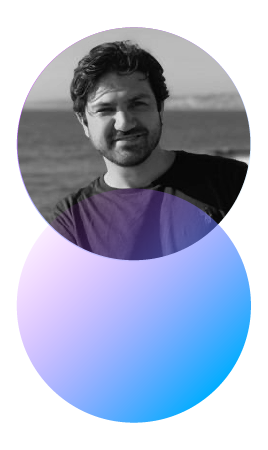
Innovation is a word that is so often misapplied and overused that it faces a risk of being rendered meaningless. Defining “innovation” is thus important to contextualizing what progress and achievement mean. In the context of life sciences organizations, the word innovation is widely used, but what does it mean in real-world applications?
In drug discovery and development, a major part of the life sciences effort, risk influences every decision, as a number of factors challenge the justifiability and profitability of investment, such as rapidly rising R&D costs, time inefficiency, low productivity, and the overwhelming likelihood of failure.
Dr.Evidence’s Chief Innovation Officer, Umut Eser, grapples with these challenges every day as part of his work leading the development of AI and machine-learning-based solutions for biomedical intelligence.
Defining innovation in life sciences
Dr.Evidence: Innovation is a broadly overused term. What does it mean to you, and more specifically for you in the context of your work?
Umut: Innovation is not a super well-defined or rigid term, and rightfully so, because it may mean a lot of different things in different contexts. At its most basic, it means that you change something that delivers huge value at the end of the day. It is highly tied to transformation and creativity. It is related to understanding, that is, in this case, figuring out market dynamics and finding solutions to problems occurring or emerging with the growth of certain technologies. In the Dr.Evidence context, innovation is about enabling the next phase of intelligence discovery. Right now the focus is using emerging technologies and organizational transformation to get to meaningful leaps forward in actionable insight.
Dr.Evidence: In the same line of thinking, can you talk about the biggest challenges or trends you see from your perspective in the life sciences generally?
Umut: Let’s focus on the drug discovery and development challenge, as it’s a huge part of the life sciences effort.
Drug discovery and development is becoming increasingly difficult and more costly, and even with the advancements we’ve seen in technology, there has been no let up from the accelerating pace of the rising costs and slowing pace. Eroom’s Law, Moore’s law in reverse and written backwards, dictates the terms of these diminishing returns: since 1950 the cost of developing a new drug roughly doubled every nine years, and in 1950 we would get 30 drugs for every one billion USD spent on R&D. Now we get less than one drug for the same investment. It’s depressing, really – if you chart a number of drugs per billion dollar investment, which is inflation adjusted, it decreases exponentially every year… and it will crash at some point because the incentive is not there to create new drugs, to create new solutions, to spend on R&D to create these therapies.
"(..)innovation is about enabling the next phase of intelligence discovery." - Umut Eser
The big challenge then is how do we reverse this? Not just decrease but reverse. We need to make it easier and cheaper to find solutions. But there are many, varied challenges in making this happen. For our purposes, one of the biggest challenges is also one of the greatest opportunities. That is, to use the information that is already out there. What is something of potential value that is exponentially increasing? The knowledge that is being deposited in the public sphere. We get new information and data every single day… we need to tap into that source of power.
Taming data overload
Dr.Evidence: This has arguably been one of the challenges, as posited by Eroom’s Law – despite the availability of ubiquitous data and relevant technology, drug discovery has continued to languish. How do you make an overwhelming volume of data manageable and, more importantly, actionable?
Umut: First of all, when you try to solve a problem, you immediately realize that browsing the literature is really challenging, time consuming, and that finding the right information requires a great deal of focus. Our first step is to make this exponentially growing body of literature easily searchable and findable … and that’s what DocSearch technology has done so far at Dr.Evidence. We already make the literature as well as real-world data and clinical trials and all sorts of information and knowledge searchable, discoverable. We need this foundational first layer to build on, and we are already there now.
The second layer is to make these findings computable. Put this into a structured format, and relate the disparate pieces of information to each other so that you not only actually look for one piece of information but also try to relate the different pieces of information from different sources easily to each other. This is where innovative ideas will germinate.
The third layer is actually drawing intelligence from these connections you’ve just made. With the intelligence you will be able to make inferences and improve the quality of decisions, which will lower the risk of failure. As we know, the failure rate is unacceptably high and is a major deterrent to development. If we can remove that barrier, we can get back on track with de novo solutions and therapies.
At the precipice of breakthroughs
Dr.Evidence: It sounds a bit like the building blocks are almost already there to some extent, but at the same time, companies face the aforementioned diminishing returns from the work they are doing. If some of these companies are already using AI and ML and still experience this inertia, we are not quite at a breakthrough insight stage yet. We still have a ways to go before we get to a place where breakthroughs that never seemed possible before are possible. Is this an accurate assessment? Are we close yet, or are we making incremental steps toward that inflection point?
Umut: When I see new fields emerging, and breakthrough innovations happening, the breakthroughs are often sitting at the cross-section of multiple emerging technologies or enablements used together. This is the direction we are headed in, and it will require incremental steps.
The first way we facilitate this move toward the inflection point is to tame the exponentially increasing amounts data. Until recently, we didn’t have good methods to handle and appropriately analyze all of this data. We had to have feature engineering, were trying to extract information by hand, and anyone interacting with the data had to have deep domain knowledge to be able to build these analytics. In addition, these tools were often developed completely independently in different areas, so the tools and insights were bespoke or siloed. Right now, we have technology, which I call representation learning technology, but often known as deep learning technology… that allows us to learn computable representations of biomedical concepts and relationships from the observed data, which is going to be used for all downstream tasks and applications.
Synthesizing complexity: Integrated data
Dr.Evidence: So you’d argue that integrating data and finding relationships through machine processes to aid human judgement is going to drive innovation forward?
Umut: You know the famous ancient Indian parable where there is an elephant and six blind people are trying to understand the elephant by each one touching one part of the elephant? Each man touches a different part trying to define what they are experiencing. The one holding the trunk says the elephant is like a snake. The one holding the tusk says the elephant is like a spear. My point here is that they are not integrating the information they have available and looking at the big picture. When we get to a point where we can integrate all the knowledge we are gathering within a single system, I believe the next layer of understanding of disease and physiology will emerge.
If you step back and look at the situation, biology is complex and the complexity requires a holistic view… and what does that mean? It is the opposite of reduction. Reductionist approaches take complexity and try to reduce to its smaller components and solve the parts to get a solution to the whole. It does not work too efficiently in biological systems … so far our drug discovery and development pipeline in pharma and life sciences has been based on this reductionist approach, what we call target identifications, mostly trying to find a responsible protein or a gene for a particular disease and try to target that disease.
Most diseases are very complicated interaction with all these biological pathways and relations which emerge at a higher level, and what we are trying to do is find a responsible single protein. This approach is getting increasingly difficult to solve, and to get there we need a collective integrated approach – all levels of observation integrated into a highly efficient system. Let’s call this a knowledge management system…. We need to integrate this into knowledge management system to be able to ask the questions and get the questions from the system with all layers of complexities.
At some point when we start to integrate different sources of information into a connected knowledge base, then we are at the point that we can jump forward. Right now the data market is fragmented, we are collecting and trying to analyze data independently from each other and are coming up short by trying to solve one piece at a time – that’s actually a step forward but we need a few more steps in order to make this happen.
Dr.Evidence: How far away does that feel, or can you estimate that?
Umut: I think it’s the next step. That is what we are building at Dr.Evidence right now. We are creating a biomedical intelligence hub to help life sciences companies, researchers, physicians, and others across the industry, to be able to get that integrated information and manage the knowledge to provide and serve intelligent predictions – actually, first of all serve existing relationships between biomedical concepts and then serve next-level predictions.
Dr.Evidence: We are experiencing an accelerating pace of data accumulation and analysis, and see the potential for this to influence outcomes and even the hypotheses, questions, and the direction the research can take. With this fast feedback loop, do the outcomes or answers that you get from those questions also feed into the way the technology develops, in a virtuous cycle manner?
Umut: In order to see a qualitative fundamental change, you often need to expect a positive feedback loop, virtuous cycle, to have a phase transition. What is the loop here? Definitely, first of all, the community-wide feedback from what is being searched, what are the trends, what is the next level of research… definitely informative, but also community-wide feedback on increasing the quality of information. So the ideal goal here is being able to integrate the knowledge – not only provide the public knowledge to companies and researchers but also be able to integrate their own internal data as well, contextualizing their internal data with the public information.
That is where we believe the feedback will come from. Our knowledge management system will provide the actionable hypotheses in order to achieve long-term goals, and the output of that is going to be fed back into the knowledge graph/knowledge management system… we would like to close the loop in that way… as a long-term active learning schema.
The bottom line is, I have been on the other side of the table as a person who worked in biotech companies trying to find solutions. What I see as most important in life sciences contexts is being able to reduce the risk involved in any project. Life sciences, pharma, biotech, all rely heavily on research – it is unlike tech companies – life sciences have to do extensive R&D and that is a vast investment and an undeniable fact. This means that they need to spend a lot of money in order to get the answers they need. If we reduce the cost of acquiring a particular answer, we can create efficiencies, reduce timelines, everything is going to be accelerated from there on.
But how do we do that? That’s the holy grail – when our knowledge management system starts providing actionable paths to answer a particular question. We are developing a solution that provides the path to get answers to questions, not an oracle that will spit out answers.
To give an example, how can I target a particular protein, or how can I target a particular disease, these types of questions do not have just one simple answer. It is complex and difficult to navigate, and we provide something like navigational tools. Let’s say you’re targeting high-level disease, type II diabetes in a particular population, let’s say, with dementia. How can I treat this unmet need? Once you identify this unmet need, you would like to identify the layers of state shifts… not just at the protein level, but also at the organelle, pathway, cellular, and systemic level state shifts that are causal to diseases. Once we identify these, the intelligence system that we provide will give a path to answer this question. It won’t say “Use this drug” – instead we are creating a solution that guides and augments the experts to achieve the solution, and makes them 10x or 100x more productive. Our AI is not looking to replace expertise, but rather widen their expertise, emphasize it and empower experts with accelerated ways to get the answers and a minimized risk for any venture that they take on.