Viewpoints
From data to insight: How advanced search, analytics and AI technologies deliver for pharmaceutical and biotech companies
Q&A with Dr.Evidence’s Head of Search, Arturo Devesa, and Senior Software Engineer for DocAnalytics, Kris Zyp
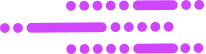
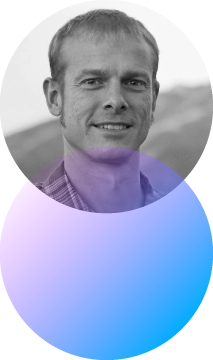
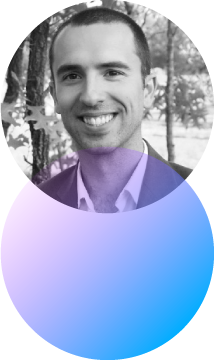
A case in point: the cost of developing a new treatment could require anywhere from an estimated USD 1.5 billion to USD 2.6 billion. With as many as nine of ten candidate therapies failing between phase I trials and regulatory approval, much of this expense is wasted. According to a study published by the Massachusetts Institute of Technology, only 13.8% of drugs successfully pass clinical trials. With these kinds of factors at play, how can data, machine learning (ML), and artificial intelligence (AI) change this equation and unlock cost efficiencies and innovation pathways?
Dr.Evidence’s Head of Search, Arturo Devesa, and Senior Software Engineer for DocAnalytics, Kris Zyp, tackle these challenges every day in developing the Dr.Evidence platform, which is designed to help simplify and streamline the search and analysis of relevant medical information to answer specific questions and gain actionable insights.
Activating the vast potential of data and evidence
Dr.Evidence: As search, analytics, machine learning (ML), and artificial intelligence (AI) technologies and techniques have advanced, companies in pharmaceuticals and biotechnology have access to a host of new tools to make their work easier. At the same time, an overwhelming amount of evidence, data, and information is available, but as is the case across industries, harnessing and identifying relevant data, and applying it to specific questions, can remain elusive and prohibitively time-consuming. How are these technologies helping these companies tap into the potential of data and evidence?
Arturo: An ongoing challenge in pharma and biotech is the sheer volume of medical literature and real-world data available, and the ever-expanding scope of that data pipeline. Evaluating these resources for relevance and value can take months and consume very large sums of money.
Kris: And this is just the first step. At the same time, all the data rushing into the system makes it clear that there are vast and varied opportunities for proactive insights, for example, in drug and disease discovery.
Add to this backdrop the pandemic, which has accelerated the need for getting immediate answers to urgent healthcare questions. The immediacy of the demand focuses a spotlight on the need for machine learning and next-generation AI technologies.
Search and analytics technology introduces the unprecedented ability to turn months-long processes into minutes-long processes instead. The Dr.Evidence platform, for example, was developed to give users actionable insights and quickly iterate through different data and meta-analyses. To be able to perform complex search and analytics functions in minutes provides an enormous time and efficiency advantage. This is always true when looked at through the lens of competitive advantage, but grows all the more important when the stakes are high and time is critical, as has been the case with Covid-19.
"We are getting near-instant answers to complex questions, but we are also finding that the answers we get are able to shape our questions and lead us to new ways of asking questions." - Kris Zyp
Dr.Evidence: Apart from how you’ve framed speed as a competitive advantage, how does the speed of the technology deliver advantages?
Kris: Speed obviously saves time, creates efficiencies, frees up resources – everything you’d expect. But the corollary to that is that because the technology’s speed provides answers quickly, we (and here we mean everyone racing to gather and understand the changing evidence landscape) discover that we can learn on the go. We are getting near-instant answers to complex questions, but we are also finding that the answers we get are able to shape our questions and lead us to new ways of asking questions. That is, the instantaneity of the insights influences the ability to form and ask different questions than a researcher started with, and creates the potential for discovering connections between questions to gain new and unforeseen insights.
For example, during the pandemic, the evidence available shifted (and the amount expanded) constantly. As the number of studies accumulated, analytics and AI tools enabled rapid re-evaluation in real-time, as the evidence itself was generated. When we consider the sheer volume of data that has been generated since the pandemic began, just within biomedical studies, we’ve seen a huge spike in published literature. Something like 600,000 articles in 1.5 years, all around coronaviruses. How would this be manageable without powerful search and analytics technologies – both in terms of time and volume?
We can see in real terms how speed and agility matter: While typical vaccines take eight to ten years to be introduced, it took 300 days from identifying the coronavirus genome to the first vaccine study.
The often difficult and time-consuming work of biomedical research can be streamlined considerably by applying search and analytics technologies. Dr.Evidence for example offers deliverables, such as the ability to search trial databases, published literature, PubMed, and a host of other sources, and the platform pulls everything together, saves your search, generates a report and can continue to actively monitor medical literature and these other sources continuously. Discovery becomes automated and integrated.
Dr.Evidence: Let’s dive a bit deeper into where you see AI fitting into this automated and integrated landscape.
Kris: AI is one of the driving forces behind how the industry is changing and how we are seeking to transform the industry. It extends the point we made earlier about changing how we ask questions and what questions we ask. Fundamentally, AI will change how we conduct research, and we want to facilitate that transformation at a deeper level. We are thinking a lot about how we integrate AI. How do we do a better job at improving human and machine interaction? AI is not a panacea in the sense that it will instantly solve a bunch of problems. Instead, it borrows ideas from how humans think and applies those concepts to improve software-based research and analysis. The human brain learns by acquiring new information and updating our knowledge or beliefs based on that learning. Software mirrors this. A common technique in AI – neural networks – borrows from how human brains work. In the development of AI, we try to rethink epistemology: how we acquire knowledge, do research, and assess its reliability, confidence in that reliability, and how to analyze or weight different parts of the analysis. Fundamentally, for users, AI does not represent a new computer with a faster processor. Instead, AI represents a change for how a user interacts with the technology or system.
Another big AI-related shift for users will be in how they research questions. That is, as we’ve stated earlier, humans will begin to learn from the machine itself. Humans learn by exploration – being able to quickly interact with data and see how different studies show different results, how different contributing factors, demographics, and other data points can change results. AI will enable more immersive experiences and engagement and interactivity with data to provide better, faster answers.
"AI may help make the future of medicine and treatment personalized at an individual level." - Arturo Devesa
Dr.Evidence: We’ve talked at a high level about how AI technology can fit into the picture, but what will ML and AI really mean or do for life sciences companies?
Arturo: AI is already transformative. But if we look at the future of finding, synthesizing and extracting actionable insight from evidence and data, tapping into the power of ML and AI will be critical.
Finding evidence and making it usable demands that we bring together an enormous amount of quantitative and qualitative data, conduct robust statistical calculations on it, and with the click of a button, provide not just the best evidence summaries available but an integrated, continuous story. That is, we move from the comprehensive literature search into advanced analytics in real-time, on the fly. Dr.Evidence is able to do this now, and ML adds predictive abilities into the mix.
Let’s say that we’re making a search query. We can use ML capabilities to predict what articles are mapped to the query we are making – helping predict an insight or connection that might not be known to humans. Humans simply do not have the capacity to generate the kinds of connections that ML can. What kinds of treatments or drugs might have been discovered ten years earlier if we’d been able to see the hidden connections?
The bottom line, of course, is that data is worthless unless you can generate insight from it. Data is worth money because people expect that insight can be extracted. Otherwise, data is just random information. With the solutions we already have, we can generate actionable insight from data. AI helps put insight into practice, moving us beyond existing use cases for medical literature and data into, for example, drug transformation (drug discovery and repurposing), identifying more relevant patient populations for clinical trials, optimizing diagnostics, tackling diseases previously seen as too difficult to take on, and so many more advanced challenges.
Yes, in the near term this could mean significant cost reductions, increased efficiencies, streamlined workflows, far less wasted time and productivity, more successful trials, and other immediately felt benefits. At the same time, though, the predictive power of AI can potentially change not just these outcomes and processes, but can change the face of medicine. For example, AI may help make the future of medicine and treatment personalized at an individual level. And we won’t get from where we are today to that level of specificity without AI integration.